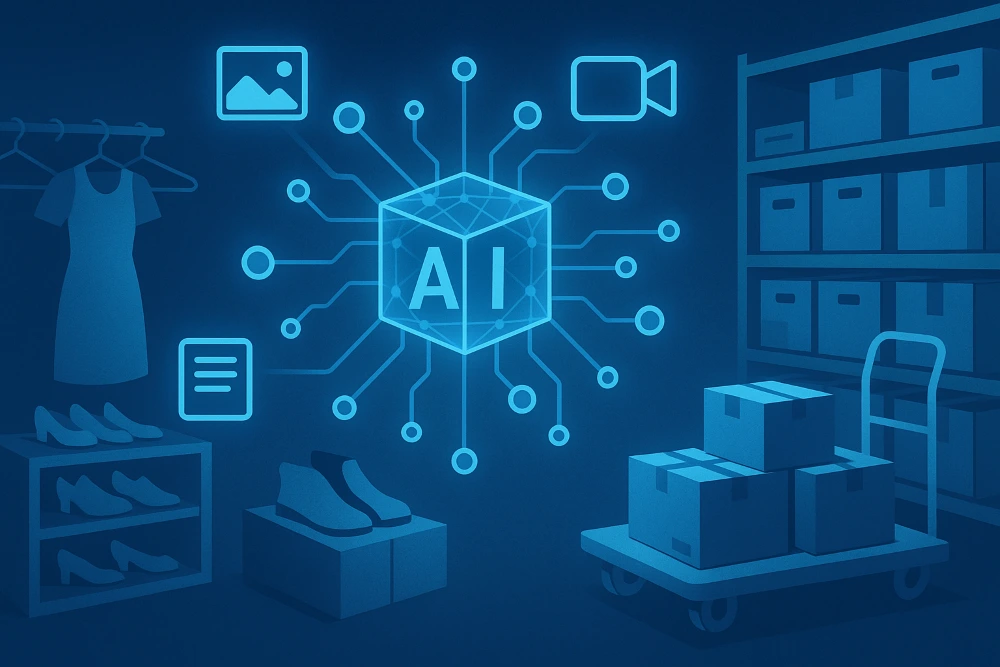
Explore how multimodal AI is revolutionizing the retail industry by enhancing personalization, inventory management, and fraud detection through integrated data insights.
The retail industry is in the midst of a transformative era, driven by the integration of multimodal artificial intelligence (AI). This cutting-edge approach leverages multiple data types — such as text, images, audio, video, and sensor data — to enable a more holistic and accurate understanding of consumer behavior, operational efficiency, and market trends. As retailers race to meet the growing demand for personalization, real-time responsiveness, and intelligent automation, multimodal AI is emerging as a cornerstone of modern retail strategy.
Understanding Multimodal AI:
Unlike traditional AI models that process a single type of input, multimodal AI combines and interprets diverse data streams simultaneously. For example, it can analyze product images, customer reviews, voice interactions, and in-store surveillance data in a unified framework. This enables AI systems to extract richer insights and make more informed decisions across various retail functions.
Key Applications in the Retail Sector:
- Personalized Shopping Experiences:
One of the most powerful applications of multimodal AI is in personalization. Retailers like Walmart and Target are leveraging multimodal models to enhance their recommendation engines. By integrating browsing history, purchase patterns, voice search inputs, and even emotional cues from video interactions, these systems are offering customers hyper-personalized product suggestions. This significantly boosts customer engagement and conversion rates, both online and in-store.
- Smart Checkout and Visual Recognition:
Retailers are deploying computer vision combined with sensor and transactional data to streamline the checkout experience. Amazon Go stores exemplify this innovation. Using a blend of camera feeds, shelf weight sensors, and customer app data, the store enables “Just Walk Out” shopping where customers pick items and leave — with payment handled automatically. This multimodal approach eliminates the need for traditional checkouts and enhances convenience.
- Â Real-Time Inventory and Supply Chain Management:
Inventory optimization is another area where multimodal AI is proving transformative. Zara, the fashion retail giant, has incorporated AI that analyzes CCTV footage, RFID sensor data, and sales records to track product movement in real time. This allows for dynamic shelf replenishment, reduced stockouts, and better forecasting, helping streamline operations and reduce waste.
- Â Sentiment and Behavioral Analytics:
Retailers are increasingly using multimodal AI to understand customer sentiment. Combining textual reviews, facial recognition, and voice tone analysis, brands can detect nuanced emotions that go beyond simple feedback scores. Sephora, for example, has begun using multimodal systems to analyze customer responses during beauty consultations, enabling more empathetic and tailored product recommendations.
- Â Fraud Detection and Loss Prevention:
Security teams are adopting multimodal AI to improve fraud detection. By fusing transactional anomalies, visual behavior cues, and biometric data, AI systems can flag suspicious activity in real time. Alibaba uses such systems in its brick-and-mortar Freshippo stores to identify theft risks and payment fraud with far greater accuracy than traditional methods.
Benefits to the Retail Ecosystem:
The integration of multimodal AI delivers tangible benefits across the retail value chain:
Enhanced Customer Engagement: Personalized and immersive experiences increase satisfaction and retention.
Operational Efficiency: Real-time insights optimize staffing, inventory, and supply chain logistics.
Improved Security: Advanced fraud detection mechanisms reduce financial and reputational risks.
Data-Driven Innovation: Unified analytics empower strategic decision-making and agile responses to market trends.
Challenges and Considerations:
Despite its advantages, implementing multimodal AI comes with challenges:
Data Privacy and Compliance: Handling biometric and behavioral data requires strict adherence to data protection regulations such as GDPR and CCPA.
Integration Complexity: Retailers must reconcile legacy systems with modern AI infrastructure, which can require significant time and investment.
Talent and Resource Constraints: Building and maintaining multimodal models necessitates expertise in machine learning, data engineering, and domain-specific knowledge.
The Future of Retail with Multimodal AI:
As technologies like 5G, edge computing, and generative AI continue to mature, the potential of multimodal AI in retail will only expand. Future applications may include:
Conversational commerce with emotionally aware virtual assistants that respond to both verbal and visual cues.
Autonomous stores with intelligent inventory systems that learn and adapt in real time.
Metaverse retail environments that combine gesture tracking, speech recognition, and eye movement analysis to create fully immersive shopping experiences.
Companies that embrace these innovations early are likely to lead in customer loyalty, operational excellence, and competitive agility.
Multimodal AI is reshaping the retail industry by enabling a deeper, more contextual understanding of consumers and operations. From personalizing customer journeys to optimizing supply chains and enhancing security, the technology is not just a trend — it’s a strategic imperative. As the digital and physical realms of retail continue to converge, multimodal AI stands at the forefront of creating smarter, faster, and more intuitive retail experiences.